Data Science and Predictive Analytics: Turning Data into Actionable Insights
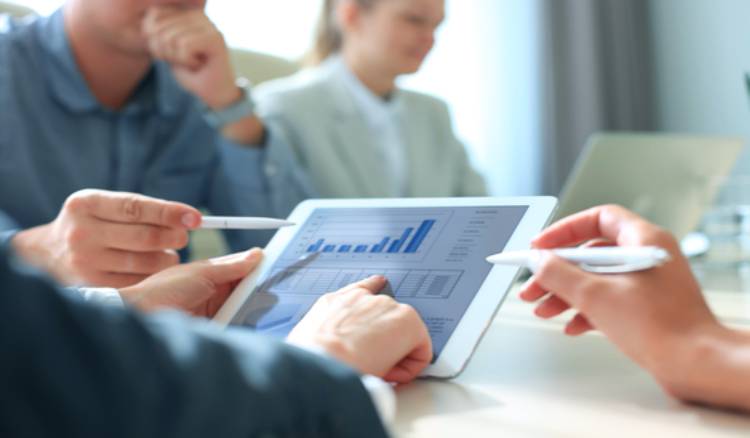
In today's digital age where data is the new currency, Data Science and Predictive Analytics are becoming indispensable tools for businesses looking to thrive in a competitive landscape. Welcome to our deep dive into these contemporary technological marvels transforming how organizations function! Our post titled "Data Science and Predictive Analytics: Turning Data into Actionable Insights" unravels the intricate process of transforming raw figures and facts into meaningful insights that drive strategic decision-making. How can we predict customer behaviour? What techniques enable us to forecast market trends? Can we anticipate operational issues before they occur? The answers lie within the domains of Data Science and Predictive Analytics. This exciting journey will exemplify how these disciplines empower businesses by giving them, not just hindsight or insight but also foresight, enabling them to stay steps ahead in their game. Prepare yourself for an intellectual adventure as we decode data like never before!
Understanding the Concept of Data Science
Understanding the concept of data science is crucial in today's digital age. Data science is the field that encompasses the collection, analysis, and interpretation of vast amounts of data to extract meaningful insights and make informed decisions. It involves the use of various statistical techniques, machine learning algorithms, and programming skills to uncover patterns, trends, and correlations within the data. Data scientists are skilled professionals who possess a deep understanding of mathematics, statistics, and computer science. They have the ability to identify relevant data sources, clean and preprocess the data, and develop and implement models and algorithms to extract actionable insights. The goal of data science is to provide organizations with a competitive edge by enabling them to make data-driven decisions, optimize business processes, and develop innovative products and services.
Role of Predictive Analytics in Data Science
Predictive analytics plays a crucial role in data science by enabling organizations to turn raw data into actionable insights. By leveraging advanced statistical algorithms and machine learning techniques, predictive analytics allows businesses to forecast future outcomes and trends based on historical data. This enables them to make informed decisions, optimize processes, and identify opportunities for growth. In the field of data science, predictive analytics helps in creating predictive models that can be used to predict customer behaviour, optimize marketing campaigns, detect fraud, and improve operational efficiency. With the increasing availability of data, predictive analytics has become an indispensable tool for businesses looking to gain a competitive edge. It empowers organizations to anticipate future trends and take proactive measures to stay ahead in today's data-driven world.
Application of Data Science and Predictive Analytics: Case Studies
The application of data science and predictive analytics has become increasingly important in various industries. This article presents case studies highlighting the potential benefits of utilizing these techniques. In the retail sector, data science and predictive analytics can help optimize inventory management and demand forecasting, allowing businesses to avoid overstocking or stockouts. In the healthcare industry, these techniques can be used to improve patient outcomes by identifying high-risk patients and implementing preventive measures. In the financial sector, data science and predictive analytics can aid in fraud detection and prevention, enabling financial institutions to protect their customers from potential losses. Moreover, in the transportation industry, these techniques can optimize route planning and improve fleet management efficiency. These case studies demonstrate the significant impact that data science and predictive analytics can have on business operations and decision-making processes.
Challenges in Implementing Data Analysis Strategies
One of the main challenges in implementing data analysis strategies is the availability and quality of data. Data scientists need access to comprehensive and accurate data to derive meaningful insights. However, often organizations face issues with data collection, integration, and cleanliness, which can hinder the analysis process. Another challenge is the complexity and volume of data. With the exponential growth of data, organizations need sophisticated tools and techniques to analyze and extract valuable insights from large datasets. Additionally, the lack of data literacy among decision-makers can pose a challenge. It is crucial for organizations to invest in proper training and education to ensure decision-makers understand the process, limitations, and potential biases involved in data analysis. Furthermore, the rapid pace of technological advancements requires data analysis strategies to be flexible and adaptable to new tools and methodologies.
The Future Outlook on Data Science and Predictive Analytics
The future outlook on data science and predictive analytics is incredibly promising. With advancements in technology and the increasing availability of big data, organizations are recognizing the immense value that can be derived from harnessing these tools. Data science and predictive analytics have the potential to revolutionize decision-making processes, enabling businesses to make more informed and strategic choices. The ability to analyze large volumes of data in real time allows organizations to identify patterns, trends, and insights that were previously undetectable. This invaluable knowledge can then be used to optimize operations, anticipate customer behaviour, mitigate risks, and drive innovation. As the field continues to evolve, we can expect to see even more sophisticated algorithms and techniques being developed, further enhancing the power of data science and predictive analytics in transforming businesses and industries.